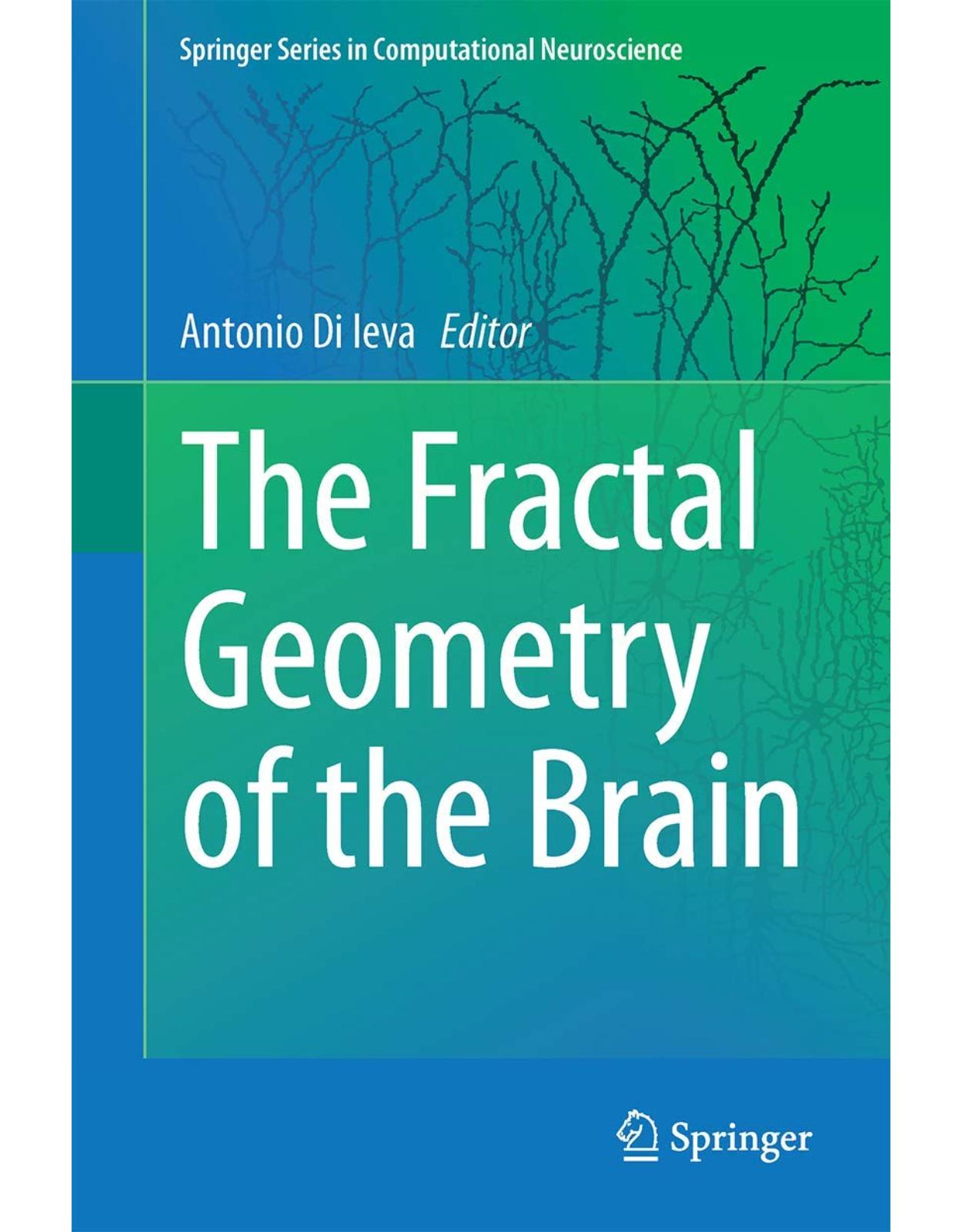
The Fractal Geometry of the Brain
Livrare gratis la comenzi peste 500 RON. Pentru celelalte comenzi livrarea este 20 RON.
Description:
Reviews the most intriguing applications of fractal analysis in neuroscience with a focus on current and future potential, limits, advantages, and disadvantages. Will bring an understanding of fractals to clinicians and researchers also if they do not have a mathematical background, and will serve as a good tool for teaching the translational applications of computational models to students and scholars of different disciplines. This comprehensive collection is organized in four parts: (1) Basics of fractal analysis; (2) Applications of fractals to the basic neurosciences; (3) Applications of fractals to the clinical neurosciences; (4) Analysis software, modeling and methodology.
Table of Contents:
Part I: Introduction to Fractal Geometry and Its Applications to Neurosciences
Chapter 1: The Fractal Geometry of the Brain: An Overview
1.1 From the Fractal Geometry of Nature to Fractal Analysis in Biomedicine
1.2 From Euclid to the Fractal Metrology
1.3 The Fractal Geometry of the Brain
1.4 Fractal Dimension and Neurosciences
References
Chapter 2: Box-Counting Fractal Analysis: A Primer for the Clinician
2.1 Fractal Analysis: What Does It Measure?
2.2 How Is a DF Calculated?
2.2.1 Practical Points
2.2.1.1 Statistical Self-Similarity
2.2.1.2 DF and Density
2.2.1.3 The DF in Neuroscience
2.3 Box Counting
2.3.1 Sampling, S, and N in Box Counting
2.3.2 Methodological Issues in Box Counting
2.3.2.1 Regression Lines
2.3.2.2 Sampling Size, Location, and Rotational Orientation Bias
2.3.2.3 Box-Counting Solutions
2.4 Lacunarity
2.4.1 Calculating Lacunarity
2.4.2 Understanding the DB and Λ
2.4.2.1 Pattern Idiosyncrasies
2.4.2.2 Applying Lacunarity
2.5 Grayscale Volumes and Box Counting
2.6 Multifractal Analysis
2.6.1 Reading the Dq Curve
2.6.2 Reading the ƒ(α) Curve
2.6.3 Applying Multifractal Analysis
2.7 Subscanning
2.8 The Validity of 2D Patterns from 4-Dimensional Reality
2.8.1 Control and Calibration
2.9 Conclusion
References
Chapter 3: Tenets and Methods of Fractal Analysis (1/f Noise)
3.1 Tenets and Methods of Fractal Analysis (1/f Noise)
3.2 Statistical Terms: Parameter, Estimator, Estimate
3.3 Properties of 1/f Noise: Self-Similarity and Long Memory
3.3.1 Memory
3.3.2 Stationarity
3.4 Fractal Parameters
3.4.1 Hurst Coefficient
3.4.2 Scaling Exponent (α)
3.4.3 Power Spectra
3.4.4 Power Exponent
3.4.5 Differencing Parameter (d)
3.5 Estimators of Fractal Parameters
3.6 Identification of Fractal Noise in Empirical Settings
3.7 Summary
References
Chapter 4: Tenets, Methods, and Applications of Multifractal Analysis in Neurosciences
4.1 Introduction
4.2 Tenets of Multifractal Analysis
4.3 Methods of Multifractal Analysis
4.3.1 Time Domain Methods
4.3.1.1 Generalized Fractal Dimensions and Multifractal Spectrum
4.3.1.2 The “Sandbox” or Cumulative Mass Method
4.3.1.3 The Large-Deviation Multifractal Spectrum
4.3.1.4 Multifractal Detrended Fluctuation Analysis: MDFA
4.3.1.5 Multifractal Detrended Moving Average: MDMA
4.3.2 Time-Frequency Domain Methods
4.3.2.1 Wavelet Transform Modulus Maxima: WTMM
4.3.2.2 Wavelet Leaders-Based Multifractal Analysis: WLMA
4.3.2.3 Multifractional Brownian Motion: mBm
4.4 Applications of Multifractal Analysis
4.4.1 Electroencephalogram Signal: EEG
4.4.2 Brain Imaging
4.5 Conclusion
References
Part II: Fractals in Neuroanatomy and Basic Neurosciences
Chapter 5: Fractals in Neuroanatomy and Basic Neurosciences: An Overview
5.1 What About the Brain?
5.2 Fractals, Neurons, and Microglia
5.3 Brains and Trees
5.4 Increase of the Fractal Dimension from “Too Smooth to Too Folded” Human Brains
5.5 Neuronal Networks
References
Chapter 6: Morphology and Fractal-Based Classifications of Neurons and Microglia
6.1 A Brief Introduction to Neurons and Microglia
6.1.1 Neuronal and Microglial Morphology in Context
6.2 Fractal Analysis of Neurons
6.2.1 Fractal Analysis of Dendritic Arbors
6.2.2 Methodological Issues
6.2.2.1 Complementary Methods
6.2.2.2 3D Analysis
6.3 Microglia
6.4 Future Directions
References
Chapter 7: The Morphology of the Brain Neurons: Box-Counting Method in Quantitative Analysis of
7.1 Introduction
7.2 Starting from the Fractal Geometry Toward the Fractal Analysis
7.2.1 Fractal Geometry in 2D Space
7.2.2 Self-Similarity and Scaling
7.2.3 Fractal Analysis
7.3 Box-Counting Method
7.3.1 Application on 2D Digital Image
7.3.2 The Software for Box-Counting
7.4 Material
7.5 Box-Counting Methodology
7.5.1 Image Size and Resolution
7.5.2 Image Rotation
7.5.3 Image Representation
7.6 Discussion
References
Chapter 8: Neuronal Fractal Dynamics
8.1 Synapse Formation from the Perspective of Molecular and Cellular Biology
8.2 Fractal Time-Space in the Dynamic Process of Synapse Formation
Appendix
8.2.1 Entropy and Dynamics of Synapse Formation in Fractal Time-Space
References
Chapter 9: Does a Self-Similarity Logic Shape the Organization of the Nervous System?
9.1 Introduction
9.2 Structural Self-Similarity of the Nervous System
9.2.1 Cell Level: Complex Geometry of Neurons and Glial Cells
9.2.2 Tissue Level
9.2.2.1 Central Nervous System
9.2.2.2 Peripheral Nervous System
9.3 A Self-Similarity Logic Drives the Functional Features of the CNS
9.3.1 Interaction-Dominant Dynamics in the CNS
9.3.1.1 The Concept of “Fringe”
9.3.1.2 The Concept of “Lateral Inhibition”
9.3.2 Remodeling Processes in the Nervous System
9.4 Concluding Remarks: A Place for Self-Similarity in a Global Model of the Nervous System?
References
Chapter 10: Fractality of Cranial Sutures
10.1 Biology of Skull Suture Development
10.2 Fundamental Principle of Fractal Structure Formation: “The Same Rule Appears on Different S
10.3 Models of Skull Suture Development
10.3.1 Eden Collision Model
10.3.2 Partial Differential Equation (PDE)-Based Model and the Koch Curve
10.3.3 Mechanics-Based Model and DLA
10.4 Future Directions
10.4.1 Other Classes of Models That Generate Fractal Structures
10.4.2 Experimental Verification of Theoretical Models
10.4.3 Fractal Suture Analysis and Craniosynostosis in a Clinical Setting
References
Chapter 11: The Fractal Geometry of the Human Brain: An Evolutionary Perspective
11.1 Introduction
11.2 Principles of Brain Evolution
11.2.1 Evolution of the Cerebral Cortex
11.2.2 Mechanisms of Cortical Folding
11.2.3 Scaling of the Primate Neocortex
11.3 Fractal Geometry of Convoluted Brains
11.3.1 Principles of Scaling
11.3.2 Fractal Scaling of the Neocortex
11.4 Fractal Principles of Neural Wiring
11.4.1 Neocortical Wiring
11.4.2 Neural Network Communication
11.4.3 Limits to Information Processing
11.5 Concluding Remarks
References
Part III: Fractals in Clinical Neurosciences
Chapter 12: Fractal Analysis in Clinical Neurosciences: An Overview
12.1 Clinical Neurology and Cerebrovascular System
12.2 Neuroimaging
12.3 Neurohistology, Neuropathology, and Neuro-oncology
12.4 Fractal-Based Time-Series Analysis in Neurosciences
12.5 Cognitive Sciences, Neuropsychology, and Psychiatry
12.6 Limitations of Application of Fractal Analysis into Clinical Neurosciences
12.6.1 The “Black Box”
References
Chapter 13: Fractal Analysis in Neurological Diseases
13.1 Geometric Fractal Analysis Applied to Neuroscience
13.2 Use of Dynamic Fractal Analysis in Neurology
13.3 Diagnostic Precision of Fractal Dimension
13.3.1 Depression and Schizophrenia
13.3.2 Alzheimer’s Disease and Autism
13.3.3 Epilepsy
13.3.4 Neural Loss in Retinal Tissue
13.3.5 Brain Tumors
13.4 Conclusion and Future Perspectives
References
Chapter 14: Fractal Dimension Studies of the Brain Shape in Aging and Neurodegenerative Diseases
14.1 Introduction
14.1.1 Anatomical Shape Features of Interest
14.1.2 Fractal Dimension Methods
14.2 Fractal Dimension Studies of the Brain Shape
14.2.1 Aging
14.2.2 Alzheimer’s Disease
14.2.3 Amyotrophic Lateral Sclerosis
14.2.4 Epilepsy
14.2.5 Multiple Sclerosis
14.2.6 Multiple System Atrophy
14.2.7 Stroke
14.3 Discussion
References
Chapter 15: Fractal Analysis in Neurodegenerative Diseases
15.1 Alzheimer’s Disease and Vascular Dementia
15.1.1 Fractal Dimension: A Classifier for the AD Pathology
15.1.2 Imaging and Fractal Analysis in AD
15.2 Other Neurodegenerative Diseases
15.3 Conclusion
References
Chapter 16: Fractal Analysis of the Cerebrovascular System Physiopathology
16.1 Introduction
16.2 Cerebral Autoregulation as a Feedback Loop
16.3 Variability and Complexity
16.4 Methodology of Variation and Fractal Analysis
16.5 Hurst Coefficient HbdSWV
16.6 Spectral Index ß
16.7 Spectral Exponent α
16.8 Fractal Analysis of Human CBF
16.9 Decomplexification
16.10 Frequency-Dependent CBF Variability
16.11 Conclusions
References
Chapter 17: Fractals and Chaos in the Hemodynamics of Intracranial Aneurysms
17.1 Introduction
17.2 Fractal Patterns in Time-Dependent Flows
17.3 Basic Concepts Demonstrated on a Simplified 2D Case
17.4 Measuring Chaotic Quantities from Residence Times
17.5 Appearance of Chaotic Flow Inside Intracranial Aneurysms
17.6 Concluding Remarks
References
Chapter 18: Fractal-Based Analysis of Arteriovenous Malformations (AVMs)
18.1 Introduction
18.2 Neuroimaging of AVMs
18.3 AVMs’ Angioarchitecture Morphometrics
18.4 Computational Fractal-Based Analyses of AVMs
18.4.1 AVMs’ Fractal Dimension
18.4.2 Fractal Dimension of the Nidus and Its Relevance in Radiosurgery
18.5 Limitations
18.6 Computational Techniques for the Automatic Nidus Identification
18.7 Conclusion
References
Chapter 19: Fractals in Neuroimaging
19.1 Introduction
19.2 Fractals in Brain Magnetic Resonance Image Classification
19.3 Other Applications of Fractal Analysis in Neuroimaging
19.4 Conclusion and Future Perspective
Appendix: Fractal Analysis Techniques
Range-Scale-Based Hurst Exponent
Detrended Fluctuation Analysis
Generalized Hurst Exponent
References
Chapter 20: Computational Fractal-Based Analysis of MR Susceptibility-Weighted Imaging (SWI) in Ne
20.1 Introduction
20.2 Technical Aspects of SW Imaging
20.3 SWI in Neuro-oncology
20.3.1 Morphometrics and Fractal-Based Analysis of SWI in Brain Tumors
20.4 Future Perspective of SWI in Neurotraumatology
20.5 Limitations
20.6 Conclusion
References
Chapter 21: Texture Estimation for Abnormal Tissue Segmentation in Brain MRI
21.1 Introduction
21.2 Background Review
21.2.1 Fractal (PTPSA) Texture Feature Extraction
21.2.2 Multi-fractal Brownian Motion (mBm) Process and Feature Extraction
21.3 Methodology
21.3.1 Preprocessing
21.3.2 Feature Extraction, Fusion, Ranking, and Selection
21.3.3 Classification with Random Forest
21.4 Results and Discussion
21.5 Conclusion and Future Work
References
Chapter 22: Tumor Growth in the Brain: Complexity and Fractality
22.1 Introduction
22.2 Fractal Dimension and Brain Tumors
22.3 The Scaling Analysis Approach
22.4 Data Time-Like Series, Visibility Graphs, and Complex Networks
22.5 Conclusions and Future Prospects
References
Chapter 23: Histological Fractal-Based Classification of Brain Tumors
23.1 Introduction
23.2 Fractal Morphometry of Tissue Complexity
23.2.1 Fractal Dimension Estimation
23.2.2 Related Work
23.3 Automated Histopathological Image Analysis
23.3.1 Image Preparation
23.3.2 Pre-processing and Focal Regions Segmentation
23.3.3 Feature Extraction and Classification
23.3.4 Qualitative Enhancement and Grading Results
23.4 Characterizing Tissue via Fractal Properties
23.5 Quasi-fractal Texture Representation
23.6 Multi-fractality Analysis
23.6.1 Assessing Fractal Texture Heterogeneity
23.6.2 Performance Under Tissue Distribution Variation
23.7 Diagnostic Challenges and Future Perspectives
23.8 Conclusion
References
Chapter 24: Computational Fractal-Based Analysis of Brain Tumor Microvascular Networks
24.1 Introduction
24.2 Brain Tumors and Vascularization
24.2.1 Immunohistochemistry (IHC)
24.3 Morphometrics of Microvascularity
24.3.1 Euclidean-Based Parameters
24.3.2 Image Analysis
24.4 Fractal-Based Morphometric Analyses of Microvessels
24.4.1 Microvascular Fractal Dimension (mvFD)
24.4.2 Local Fractal Dimension and Local Box-Counting Dimension
24.5 Fractal-Based Analysis of the Angio-Space in Brain Pathology
24.6 Limitations
24.7 Future Perspectives and Conclusion
References
Chapter 25: Fractal Analysis of Electroencephalographic Time Series (EEG Signals)
25.1 Introduction
25.2 Nonlinearity and Nonstationarity
25.3 Fractal Analysis of EEG
25.4 Examples of Application of Fractal Analysis to EEG Signals
25.4.1 Seasonal Affective Disorder: Artifacts in EEG May Be Important for Diagnosis
25.4.2 Sleep Staging: One May Analyze Raw EEG Data Without Artifact Elimination
25.4.3 Influence of Electromagnetic Fields: Comparing Qualitative Features of Df(t)
25.4.4 Epileptic Seizures and Epileptic-Like Seizures in Economic Organisms
25.4.5 Psychiatry: Assessing Effects of Electroconvulsive Therapy
25.4.6 Anesthesiology: Monitoring the Depth of Anesthesia
25.5 Conclusions
References
Chapter 26: On Multiscaling of Parkinsonian Rest Tremor Signals and Their Classification
26.1 Introduction
26.2 Multifractal Detrended Fluctuation Analysis for Nonstationary Time Series
26.3 Evidence of Multiscaling in Parkinsonian Rest Tremor Velocity Signals
26.4 Concluding Remarks and Future Research Perspectives
References
Chapter 27: Fractals and Electromyograms
27.1 Introduction
27.2 Surface Electromyogram (sEMG)
27.2.1 Generation of sEMG
27.2.2 Factors That Influence sEMG
27.3 Fractal Analysis of sEMG
27.3.1 Self-Similarity of sEMG
27.4 Method to Determine Fractal Dimension
27.5 Computation of Fractal Dimension Using Higuchi’s Algorithm
27.6 Relation of FD to sEMG
27.7 Age-Related Decrease in Fractal Dimension of Surface Electromyogram
27.8 Summary
References
Chapter 28: Fractal Analysis in Neuro-ophthalmology
28.1 Eye and Nervous System
28.2 Retinal Microvascular Networks and Ophthalmopathies
28.3 Our Experience: Neuro-ophthalmological Disorders
28.3.1 Optic Neuritis Versus Nonarteritic Anterior Ischemic Optic Neuropathy: Retinal Microvascular
28.3.1.1 Patients
28.3.1.2 Image Analysis
Entropy, D1
28.3.1.3 Statistical Analysis
28.3.1.4 Results
28.3.2 Sjogren’s Syndrome: Corneal Nerve Plexus
28.3.2.1 Patients
28.3.2.2 Image Analysis
Geometric Complexity, D0
28.3.2.3 Statistical Tests
28.3.2.4 Results
28.4 Discussion
References
Chapter 29: Fractals in Affective and Anxiety Disorders
29.1 Introduction
29.2 Fractals and Affective Disorders
29.3 Fractals and Anxiety Disorders
29.4 Fractals in Affective and Anxiety Disorders Treatments
29.5 Conclusions
References
Chapter 30: Fractal Fluency: An Intimate Relationship Between the Brain and Processing of Fracta
30.1 Introduction: The Complexity of Biophilic Fractals
30.2 Fractal Fluency
30.3 Enhanced Performance and Fractal Aesthetics
30.4 Conclusion: The Brave New World of Neuro-Aesthetics
References
Part IV: Computational Fractal-Based Neurosciences
Chapter 31: Computational Fractal-Based Neurosciences: An Overview
31.1 How to Compute Fractals in Clinical Neurosciences
31.2 Fractals in Bioengineering and Artificial Intelligence
31.3 Conclusive Remarks: Towards a Unified Fractal Model of the Brain?
Chapter 32: ImageJ in Computational Fractal-Based Neuroscience: Pattern Extraction and Translation
32.1 Introduction
32.2 What Is ImageJ?
32.2.1 Removing Barriers with Free, Open-Source Software
32.2.2 Shaping Computational Fractal-Based Neuroscience
32.2.2.1 Making Fractal Analysis Accessible and Customizable
32.3 Where Does IJ Fit in Fractal-Based Neuroscience Today?
32.4 Pattern Extraction
32.4.1 Pattern Types
32.4.2 Extraction Methods
32.4.2.1 Built-in Functions
32.4.2.2 Tracing Plug-Ins
32.4.2.3 Thresholding
32.4.2.4 Customized Pattern Extraction Methods
32.5 Conclusion
References
Chapter 33: Fractal Analysis in MATLAB: A Tutorial for Neuroscientists
33.1 MATLAB Packages and Toolboxes for Fractal Analysis
33.2 MATLAB Examples: Fractal Dimension Computation for 1D, 2D, and 3D Sets
33.2.1 EEG Fractal Dimension
33.2.2 Brain MRI Fractal Dimension of the Gray Matter with FracLab
33.2.3 Fractal Dimension Computation of an MRI Volume of the Brain White Matter with a Boxcoun
33.3 Other Software and Online Resources for Fractal Analysis
33.4 Conclusions
References
Chapter 34: Methodology to Increase the Computational Speed to Obtain the Fractal Dimension Usin
34.1 An Introduction to GPU Programming
34.1.1 NVIDIA CUDA
34.1.2 OpenCL
34.2 Previous Work
34.3 Box-Counting Algorithm
34.4 GPU Implementation
34.5 Results
34.5.1 Hardware and Test Models
34.5.2 Implementation Results
34.6 Discussion, Conclusions, and Future Work
References
Chapter 35: Fractal Electronics as a Generic Interface to Neurons
35.1 Introduction
35.2 Fabrication of the Fractal Interconnects
35.3 Functionality of the Fractal Interconnects
35.4 The Biophilic Interface
35.5 Conclusions
References
Chapter 36: Fractal Geometry Meets Computational Intelligence: Future Perspectives
36.1 Introduction
36.2 Fractal Analysis and Brain Complexity
36.3 Computational Intelligence Methods and the Challenge of Processing Non-geometric Input Space
36.4 On the Interplay Between Fractal Analysis and CI Methods
36.5 Future Perspectives and Concluding Remarks
References
Erratum to: The Fractal Geometry of the Brain
Index
An aparitie | 2016 |
Autor | Di Ieva |
Dimensiuni | 15.6 x 3.33 x 23.39 cm |
Editura | Springer |
Format | Hardcover |
ISBN | 9781493939930 |
Limba | Engleza |
Nr pag | 607 |
Clientii ebookshop.ro nu au adaugat inca opinii pentru acest produs. Fii primul care adauga o parere, folosind formularul de mai jos.